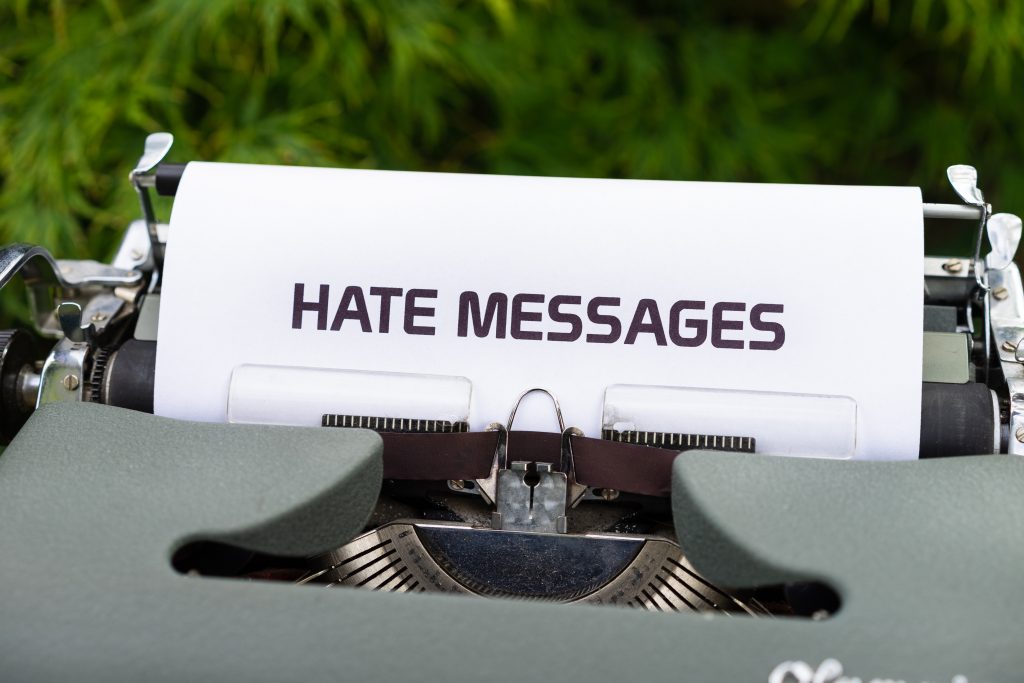
For the past month, I was participating in Rewire’s abusive speech detection project in the role of entries annotator. Its been an amazing last couple of weeks in which I learned more about what such a project looks like from the inside and was able to meet very interesting individuals from the NLP field.
About Rewire
Rewire is a start-up company established by researchers from Alan turning Institute, Bertie Vidgen and Paul Röttger who were both involved in similar projects before deciding to start their own company. Rewire is delivering socially responsible AI solutions for online safety. Their proprietary algorithm is trained for various problems ranging from hate speech to sexism detection or in this case, abuse identification.
Why Have I participated?
I learned about this project through one of my colleagues at the Social Informatics Research Group and since it was related to my current research, I decided to apply. I wanted to learn more about what such a project looks like from the inside, explore the team’s working dynamics and what could be expected from the participants. On top of that, I was hoping to meet people from the AI field so that I could incorporate some of their expertise into my own work.
How did it go?
Throughout the whole duration of the project, I enjoyed the working environment created by the company. From the beginning, we were given a clear picture of what the whole project will look like and what is expected from us. I was surprised by how much our input was considered in the entire annotation process. We were given a chance to discuss harder cases and could always count on help from the expert annotators, providing explanations on top of the answers. That way the entire process run smoothly and deadlines could be easily met. I have to say that the Rewire team was really flexible when it came to the working conditions which made working for them an amazing experience. Moreover, we were also given a chance to discuss the results and any doubts during weekly meetings which gave the team the feeling of being involved in the process, thus resulting in greater productivity (in my opinion).
During the project, I learned how severe the issue with abuse on the internet really is. After the first batch of entries, I immediately understood the importance of such work. The issue is very severe and users are getting more sophisticated in creating their abusive entries in a way that the traditional safety systems won’t work. Working towards something with a positive impact on the agenda gave me the motivation to excel at the job.
What Have I learned?
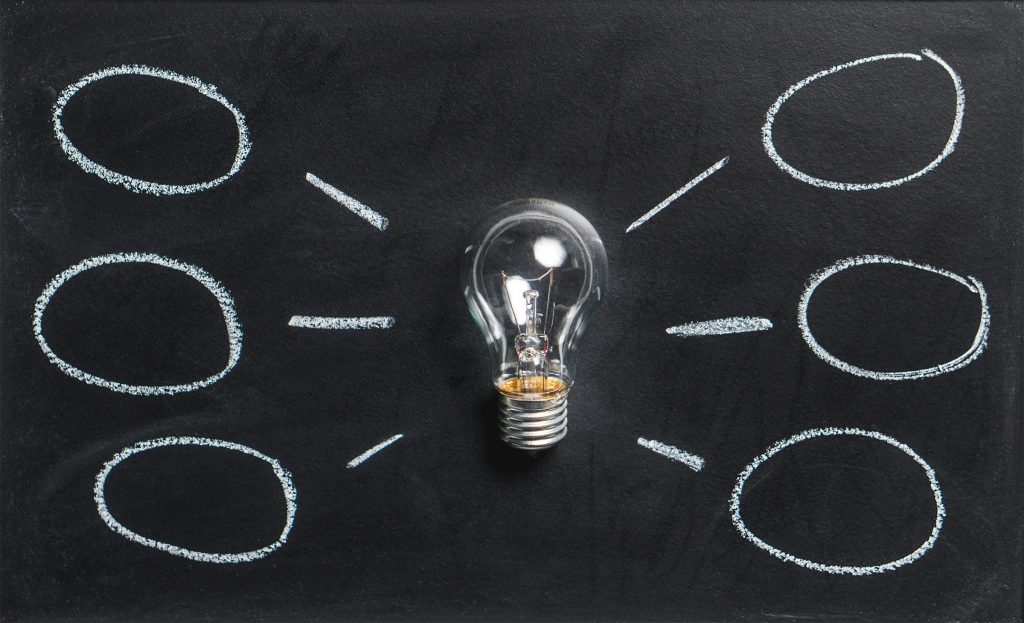
First of all, I explored how such a project progresses. If I were to use a similar method in the future for my research or even my PhD I now know where to start, what tools can I use for the communication and what is the remuneration for such task. The knowledge I gained will definitely help me set up a similar task in the future and made me more confident about working in a large team environment.
As for my second objective, I was able to establish contact with the company’s CTO Paul Röttger, who was keen enough to organise a meeting to discuss the algorithms used by a company as well as share some insights regarding the project itself as well as his personal experience in the field. Hopefully, I would be able to discuss my work with him and will be given an opportunity to hear feedback from the experienced AI expert. I want my research to be most impactful for the LM experts, policymakers and most importantly job seekers. To do that I need to ensure that all of the inefficiencies are addressed accordingly and that the methods I use will lead to the utmost results. Having a chance to speed up with someone with this experience in such early stages of research is extraordinary and likely to improve the entire process.
Thank you Rewire for such a great experience and looking forward to your future projects!